While data scientists have many resources in their tool belt, our research shows that proficiency with data mining and visualization tools consistently ranks as one of the most important skills in determining project success.
We used two methods to rank data science skills. The first way was based on the frequency with which professionals possessed the skills. This method identified data science skills that are common across data scientists. The second way based on the correlation between the data scientists’ proficiency in the skill and project outcome. This method identified data science skills that are linked to project success. Comparing the results using these two ranking methods lead to some interesting conclusions about specific data science skills. Over the next few weeks, I will be exploring specific data science skills and what these findings mean to data scientists and businesses that hire them.
Data Mining and Visualization Tools
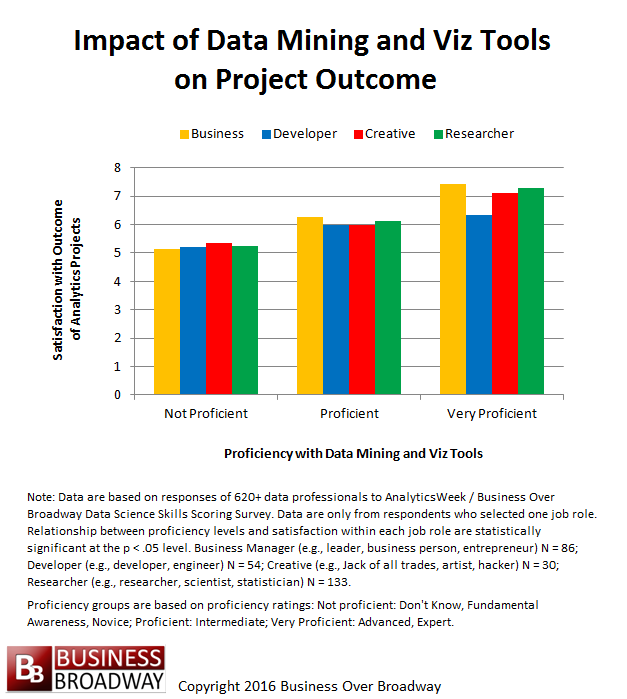
Figure 1. Relationship between Proficiency in Data Mining and Visualization Tools and Project Outcomes
We found that, across all the data professionals we surveyed, the data science skill that had the highest correlation with project success was Data Mining and Visualization Tools. That is, data scientists who were very proficient using these tools were significantly more satisfied with outcome of their work compared to data scientists who were not proficient using these tools.
Even when we examined the data science skills for each of the four data roles (i.e., business, developer, creative, researcher), being proficient in Data Mining and Visualization Tools was ranked among the top five data science skills for each job role (Business: ranked 2; Developers: ranked 4; Creative: ranked 2; Researcher: ranked 2). Figure 1 illustrates the difference between data scientists who are proficient in data mining and visualization tools and those who are not. The results showed that the difference between data scientists who are not proficient in data mining and visualization and those who are proficient held up for each data science role (although this difference was smaller for Developers).
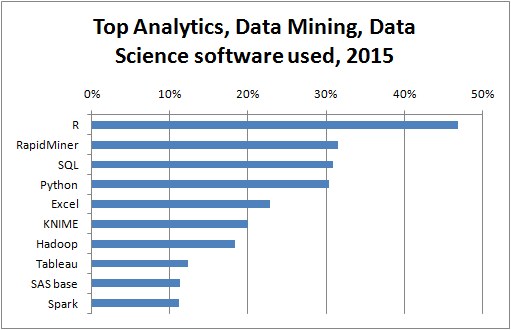
Figure 2. Popular Data Mining Tools. From 2015 KDNuggets poll.
When data professionals are not proficient using these tools, their satisfaction with the outcome of projects falls in the mid-point of the satisfaction scale (around 5.0; satisfaction scale was 0 – Extremely Dissatisfied; 5 – Neither Satisfied nor Dissatisfied; 10 – Extremely Satisfied). When data scientists are proficient in these tools, they are significantly more satisfied with the outcome of their projects.
Summary
Data become valuable when you do something with them, analyze them, visualize them. To get insight from those data, you need to need to know what the data are telling you. While other data science skills are very important in the process of extracting value from data (e.g., data integration, data cleansing), the results show that a data scientist’s ability to use tools to mine and visualize data is essential to project success. Figure 2 list some popular data mining tools from a 2015 KDNuggets poll of data professionals. Popular data mining tools include R, RapidMiner, Spark and even Excel. The infographic in Figure 3 lists some popular visualization tools.If you’re a budding data scientist, it doesn’t matter if you are a Developer or Researcher or any other any other type of data scientist. You would benefit greatly if you learn tools that help you mine and visualize your data; the more proficient in these tools you become, the better you will feel about the outcome of your analytics projects. If you are a recruiter or organization seeking new data scientists, screening candidates should include a question about data their use of data mining and visualization tools. The more your data science team members possess the skills to analyze and visualize their data, the greater success you’ll achieve in your data science projects.
Perhaps visually oriented practitioners are more optimistic &/or simply rank project outcome more highly than those who are not. Is your project success criterion subjective?