A study of 80+ companies showed that analytical leading companies (those who use analytics to gain a competitive advantage), more so than analytical lagging companies, leverage their data differently. Analytical Leaders focus their analytics to improve customer loyalty while Analytical Laggards focus their efforts primarily to reduce enterprise costs. Additionally, Analytical Leaders, compared to Analytical Laggards, tend to have more analytically-savvy customer experience and customer success programs. Analytical Leaders’ customer programs integrate customer program data with other data sources, use machine learning to gain insight, have access to data scientists and conduct in-depth research. To see the results regarding all 25 customer analytics practices, you can download the entire report for free here.
We recently released a best practices study that looked at the degree to which companies incorporated 25 practices in their customer programs (e.g., primarily customer experience or customer success programs). In the report, Customer Analytics Best Practices, we showed that analytics leading companies (those who are able to use analytics to gain a competitive advantage) adopted specific practices in their customer programs that aid in the data-to-insights value chain. In this post, I will present some of the results of that study, focusing on the adoption of research practices (e.g., machine learning, data science, data integration, data platforms, in-depth studies).
Analytics Maturity: Leaders, Challengers and Laggards
In the study, companies were segmented into three groups based on how well they use analytics to create a competitive advantage. Segmentation was based on the response to the following question: How satisfied are you with your company’s use of analytics to give them a competitive advantage? (0 – Extremely Dissatisfied to 10 – Extremely Satisfied); 0-3 = Analytical Laggards, 4-6 = Analytical Challengers; 7-10 = Analytical Leaders.
Analytical Leaders Focus on Generating Customer Insights
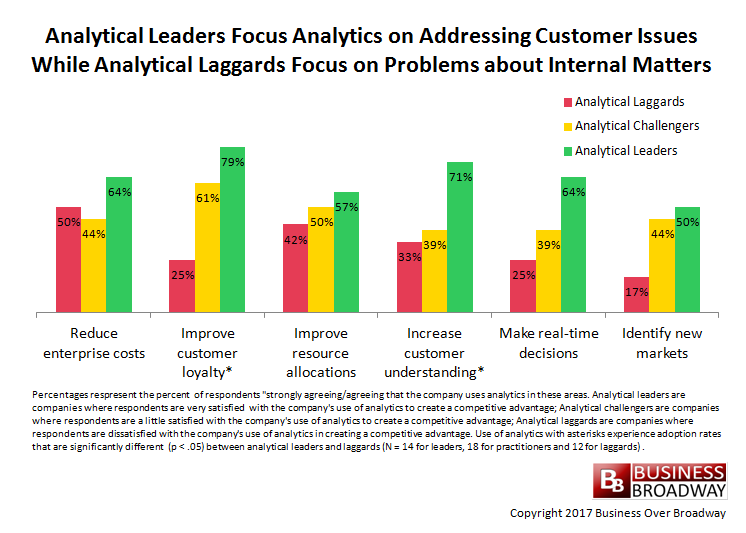
Figure 1. Analytical Leaders focus analytics efforts on understanding customers. Analytical Laggards focus analytics on improving internal matters. Click image to enlarge.
Companies can use analytics for a variety of different reasons. We asked respondents how their company primarily uses analytics. Results showed that analytical leaders use analytics differently than analytical laggards. Generally speaking, the top use of analytics for Analytical Leaders was to generate customer insights while the top use of analytics for Analytical Laggards focused on internal matters (see Figure 1). Specifically, Analytical Leaders use analytics primarily to improve customer loyalty (79%) and to increase customer understanding (71%). Analytical Laggards, however, use analytics primarily to reduce enterprise costs (50%) and improve resource allocation (42%).
Analytical Leaders Infuse Data Science Principles, Machine Learning Capabilities throughout their Customer Program
Customer-focused research using advanced analytics techniques can provide additional insight into the needs of the customer base and increase the overall value of the customer program. This type of research extends well beyond the information that is gained from the typical reporting tools that summarize customer metrics with basic descriptive statistics. Now, business leaders are able to leverage predictive analytics to help them predict future events so that they can capitalize on opportunities and mitigate risk.
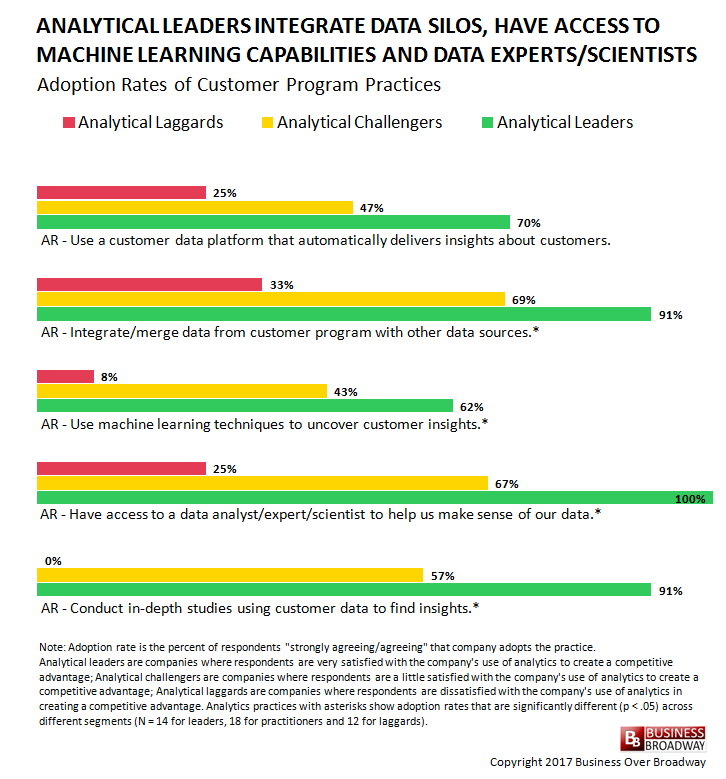
Figure 2. Analytical Leaders have customer programs that integrate data silos, have access to machine learning capabilities and data scientists.
We found that Analytical Leaders are much more analytically savvy in their customer programs compared to their counterparts (see Figure 2). Specifically, analytics leading companies, compared to analytics lagging companies, show significantly greater adoption of the following practices in their customer experience/success program:
- Integrate/merge disparate data silos with customer metrics
- Use machine learning to uncover customer insights
- Have access to a data analyst / expert / scientists to help make sense of data
- Conduct in-depth applied research using customer data to find insights
Summary: Improve Your Customer Program Using Analytics Best Practices
Our study revealed that companies’ customer experience and customer success programs would greatly benefit from the adoption of data science practices, including data integration, machine learning and deep-dive research. We found that Analytical Leaders (those businesses that gain a competitive advantage using analytics) use more sophisticated analytics methods in their customer programs compared to Analytical Laggards. Analytics leading companies focus their analytics efforts on gaining deeper customer insights and have customer programs that are equipped to glean insights from their data. Additionally, Analytical Leaders:
- Integrate data from the customer program with other data sources. Business leaders need a unified, integrated picture of their customers. Having access to all data that are relevant to your customers benefits all stakeholders. Front-line heroes are better equipped to handle individual customer needs. Additionally, data professionals are able to build better (more accurate) predictive models when they have all the relevant data. One approach to unifying your data is to leverage a customer data platform (CDP). Customer data platforms employ predictive analytics and machine learning to help surface customer insights. Also, CDPs utilizes APIs to other platforms that help support retention, lifecycle marketing and re-engagement marketing programs; that is, the insights you gain from a CDP can be integrated into your existing sales, marketing and support workflows.
- Ensure customer program teams have access to a data analyst/expert/scientist. Customer programs necessarily deal with a lot of different types (structured and unstructured) coming from many different sources (e.g., surveys, CRM, sales, support). Because customer professionals do not have the necessary skills in statistics and math, providing them access to data experts/scientists will improve their chances of making sense of and getting value from their data. These data professionals can apply the right type of analytics to surface insights that can help answer important business questions. Without these data skills, companies may be missing important insights.
- Use machine learning techniques to uncover customer insights. When customer professionals have integrated all the disparate data silos, they can be overwhelmed with the number of variables they must manage and analyze. Even if they have access to seasoned data professionals, these data pros are only human. They are simply unable to manually and quickly sift through the sheer volume of data to find optimal predictive models. Instead, to create the best predictive models, customer professionals can rely on the power of machine learning to quickly and accurately uncover the underlying insights. Machine learning algorithms continually learn, and the more data they ingest, the better they get. Coupled with the processing capability of today, these algorithms, compared to humans, can provide customer insights quickly.
To improve the effectiveness of your customer experience or customer success programs, you must be able to make sense of the data that the programs generate. An understanding of your customers now requires the infusion of advanced analytical approaches and methods into your customer program. Figure 3 summarizes the major differences across the levels of analytics maturity.
Companies that have customer programs that focus on improving customer loyalty, leverage data integration, use data science professionals and adopt machine learning capabilities will have a competitive advantage over their analytics-challenged counterparts.
Hi,
Re: the three best practice advice points above.
All 3 are currently being achieved on one platform called Wallscope. Wallscope are based in Edinburgh Scotland. I do not work for Walscope but if you take up the option of one of there demos then tell David and Ian I said Hi. http://www.wallscope.co.uk
You will find Wallscope interesting.
Regards