I gave a talk last week on Big Data and Customer Experience Management and how Big Data will change how companies think about their Customer Experience Management programs. This talk was part of a larger webinar on competitive analytics that was co-sponsored by TCELab and Omega Management. Some of the content below appears on prior blogs but not in the format below. When the webinar becomes available online, I will update this blog post with the link.
——————–
I think of Big Data as an amalgamation of different areas that help us try to get a handle on, insight from and use out of large, quickly-expanding, diverse data. To me, Big Data refers to the idea that companies can extract value from collecting, processing and analyzing vast quantities of data. In fact, McKinsey and Company, in a report from October), concluded that businesses who can get a better handle on these data will be more likely to outperform their competitors who do not.
Three Vs of Big Data
When people talk about Big Data, they are typically referring to three characteristics of the data: 1) Volume, 2) Velocity and 3) Variety. First, the amount of data being collected is massive. Hardware companies use sensors to collect data about the performance of their solutions housed in their clients’ company. Second, the speed at which data are being generated/collected is very fast (consider the streams of tweets). Finally, companies need to deal with different types of data, both structured and unstructured data.
Big Data Landscape
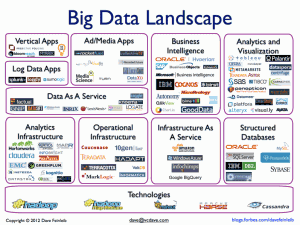
Figure 1. Big Data Landscape image was created by Dave Feinleib (dave@vcdave.com; blogs.forbes.com/davefeinleib); We have modified the image to show the reader where TCELab fits into the Big Data space. The original image can be found here.
Because extremely large data sets cannot be processed quickly using conventional database systems, companies have created new ways of processing (e.g., storing, accessing and analyzing) this big data. Data can be housed on multiple servers for quick access and employing parallel processing of the data (rather than following sequential steps).
The graphic in Figure 1, illustrates the major players in the Big Data field. At the bottom, we have the technology companies who have developed ways of processing massive amounts of data quickly. At the next level, we have infrastructure companies who provide a platform on which to integrate Big Data technology into companies’ current infrastructure. At the top of the graphic, we have the Big Data companies who help provide insight into the business data where they use science, predictive modeling and other techniques to solve a specific problem. So, it is important to be clear when talking about Big Data companies.
Three Big Data Approaches
Brian Gentile, CEO of Jaspersoft, argues for a solution-oriented approach to understanding the value of Big Data. Before selecting a vendor, you first need to understand the problem you are trying solve. Keep in mind that Big Data is not just about analyzing data quickly; it is about analyzing data intelligently, perhaps with some theory-driven analyses. Gentile’s three Big Data approaches include:
- Interactive Exploration – good for discovering real-time patterns from your data as they emerge
- Direct Batch Reporting – good for summarizing data into pre-built, scheduled (e.g., daily, weekly) reports
- Batch ETL (extract-transform-load) – good for analyzing historical trends or linking disparate data sources based upon pre-defined questions. Sometimes called data federation, this approach involves pulling metrics from different data sources for purposes of understanding how all the metrics are related (in a correlation sense) to each other.
So, be sure to understand that Big Data is not just about quick analysis of your data. It is also about integration of different sources of data. I will come back to this notion of data integration, or Batch, ETL, later in the post.
Value from Analytics
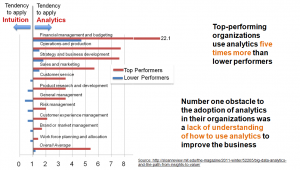
Figure 2. Businesses get value from their data using analytics. The graph was recreated for presentation. The original image can be found here.
In a late 2010 study, researchers from MIT Sloan Management Review and IBM asked 3000 executives, managers and analysts about how they obtain value from their massive amounts of data. They found (see Figure 2) that organizations that used business information and analytics outperformed organizations who did not. Specifically, these researchers found that top-performing businesses were twice as likely to use analytics to guide future strategies and guide day-to-day operations compared to their low-performing counterparts.
The MIT/IBM researchers, however, also found that the number one obstacle to the adoption of analytics in their organizations was a lack of understanding of how to use analytics to improve the business. There are simply not enough people with Big Data analysis skills. In fact, McKinsey and Company estimated that the US faces of huge shortage of people who have the skills to understand and make decisions based on the analysis of big data.
The MIT/IBM researchers also found that six out of 10 respondents cited innovating to achieve competitive differentiation as a top business challenge. Additionally, the same percentage of respondents also agreed that their organization has more data than it can use effectively. Clearly, companies want to differentiate themselves from the competition yet are unable to effectively use their data to make that happen. I think the primary Big Data problem is one of applying appropriate analytic techniques to business data.
Customer Experience Management
Customer Experience Management (CEM) is the process of understanding and managing customers’ interactions with and perceptions about the company/brand. The goal of CEM is to improve the customer experience in order to optimize customer loyalty. Top businesses who have implemented CEM programs realize that these programs can be data intensive, generating millions of data points about their customers’ attitudes, online behaviors, and even their interactions with a given employee, just to name a few. To optimize the value from these data, companies need to apply appropriate analytics to provide insights about how to increase customer loyalty.
Disparate Sources of Business Data
CEM practitioners rely heavily on customer feedback as the source of their insights, yet businesses have many different sources of business data that could provide additional customer insight (see Figure 3). These data sources fall into these general buckets:
- Operational
- Financial
- Constituency (includes employees, partners)
- Customer Feedback
Big Data federation principles can be applied to these disparate data sources to improve your ability to extract insights about the reasons behind customer loyalty and sustained business growth.
Data Integration is Key to Extracting Value
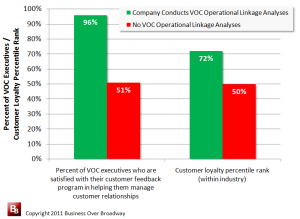
Figure 4. Companies who integrate operational data with their customer feedback get the insight that drives customer loyalty
In my research on best practices in customer feedback programs, I found that the integration of other sources of business data with customer data is necessary for an effective CEM program. As you see in Figure 4, companies who integrate operational measures with customer feedback (those are the green bars) were more satisfied with their customer feedback program and had higher customer loyalty rankings in their industry compared to companies who did not integrate those data.
Linkage Analysis
Integrating different business metrics to understand how they relate to each other is sometimes referred to as the process of Business Linkage Analysis. How you integrate/link your different metrics depends on the problem you are trying to solve or the question you are trying to answer.
For example, here are three popular questions that can be addressed using linkage analysis of disparate data sources.
- What is the $ value of improving customer satisfaction/loyalty?
- Which operational metrics have the biggest impact on customer satisfaction/loyalty?
- Which employee/partner factors have the biggest impact on customer satisfaction/loyalty?
Each question requires different datasets, merged at the right level for the appropriate analysis.
Integrating your Business Data
Figure 5 illustrates some common ways companies integrate disparate data sources. The columns represent the different types of customer feedback sources and customer metrics. The rows represent the other data sources and metrics: financial, operational and constituency. Even though many different data sources can be integrated, I refer to this approach as a “customer-centric” approach because the data are organized to gain insight about the causes and consequences of customer satisfaction/sentiment/loyalty.
Depending on the question are you trying to answer, you will use a combination of different sources of data. For example, when dealing with questions around financial metrics, you can integrate those with customer feedback at the relationship level via relationship surveys and social media sources. For operational or constituency-related question, you will need to consider other data sources and integration level (e.g., link data at transaction level instead of customer level).
How Big Data can Advance Customer Experience Management
Next, I am going to conclude by talking about how Big Data can transform the field of customer experience management. I think Big Data principles can advance the field of customer experience management space in three important ways.
1. Ask/Answer Bigger Questions
First, I think that the application of Big Data principles can help you ask and answer bigger questions about your customer. A successful CEM program is designed to deliver a better customer experience which translates into a more loyal customer base. The source of data in most CEM programs is gathered through customer feedback tools like surveys and social media sites. Businesses gain customer insight primarily by analyzing customer feedback data with little or no regard for other data sources. By linking disparate data sources to their customer feedback data, companies gain insight about their customers that they could not achieve by looking at their customer feedback data alone.
Businesses can now ask, and, more importantly, answer these types of questions.
- Where do we set operational goals in our call centers (e.g., number of handoffs, length of wait time) to ensure we maximize customer satisfaction?
- How many hours of training do employee need to ensure they can satisfy their customers?
- Which call center metrics are the key determinants of customer satisfaction with the call center experience?
- Where do we need to invest in our employee relationship (e.g., across the employee experience touch points) to ensure they deliver a great customer experience?
- Do customers who report higher loyalty spend more than customers who report lower levels of loyalty?
Companies who integrate their business data to understand the correlates of customer satisfaction and loyalty can better answer these questions and, consequently, be in a better position to effectively allocate resources in areas that they know will help improve the customer experience and maximize customer loyalty and business growth.
2. Build Company around Customers
Next, Big Data principles can help you create a customer-centric culture. By integrating different sources of business data and uncovering insights about a variety of different metrics, you build interest across different organizations in understanding what is important to the customers. The integration of different business data would necessarily involve key stakeholders from each organization, and the mere act of integration would be a catalyst for further cross-organizational discussions about the customer. Applying a customer-centric data federation and aggregation approach to business data integration helps senior leaders understand how their organization (and its metrics) impacts the customer.
Additionally, the results of customer research become more applicable to other organizations or departments when their data are used. Expanding the use of customer data to other departments (e.g., HR, Call Center, and Marketing) helps the entire company improve processes that are important to the customer. Here are some examples of how companies are using this type of research to build a customer-centric culture.
- Identifying and building customer-centric operational metrics for executive dashboards
- Removing the noise from executive reports by including only customer-centric business metrics (known to be predictive of customer satisfaction)
- Integrating customer feedback into operational systems (CRM) so front-line employees understand the interactions and attitudes of their customers
- Conducting in-depth customer research using all business data to continually gain customer insight to establish a competitive advantage
Big Data technologies and processes can go a long way in helping you support your CEM program. By taking a customer-centric approach to your Big Data, you will be able to literally build the company (its data) around the customer.
3. Predict Real Customer Loyalty Behaviors
Despite the existence of objective measures of customer loyalty (e.g., customer renews contract, recommends you, buys more), CEM programs rely on customer surveys as a way to assess customer loyalty. Measures of customer loyalty typically take the form of questions that ask the customer to indicate his or her likelihood of engaging in specific types of behaviors, those deemed important to the company/brand.
CEM professionals (me, too) typically use these self-report measures as our only measure of customer loyalty when analyzing survey data. While these loyalty metrics do provide reliable, valid and useful information, you are always interested in what customers really do. By linking up financial data and customer feedback data, you would be able to understand how the customer experience impacts real customer loyalty behavior using objective metrics, like purchase amount, products purchased, products liked, products shared and renewed contract.
End-of-quarter financial reports include customer loyalty metrics (e.g., churn rates, ARPU, repurchase rates) with no information about the factors that might impact those numbers. Traditionally analyzed at the end of the quarter as standalone metrics, these objective loyalty metrics provide no insight about how to improve them. Linking satisfaction with the customer experience to these objective loyalty measures, however, lets you build predictive models to help you understand the reasons behind your financial metrics. This is powerful stuff.
Summary
Big Data is about maximizing the value of your data. Companies that take a customer-centric approach to integrating and analyzing their business data have higher customer loyalty rankings than companies who do not. For business, the way to maximize the value of their business data is to understand how customer satisfaction and loyalty are related to different business metrics.
Big Data integration can help improve the field of customer experience management by allowing you to ask and answer bigger questions about customers. Additionally, integrating disparate data sources with customer feedback is a great way to support cross-departmental collaboration on customer research. Finally, Big Data will allow us to develop and use predictive models of real loyalty behaviors and financial outcomes instead of just self-reported loyalty behaviors. The value of your CEM program to your company and, perhaps your career, will improve greatly when the program can be linked to real, measureable financial outcomes.
Companies can gain deep customer insight through a Big Data integration approach. There is no doubt that Big Data will impact the CEM space. The field of CEM, however, can also help shape the Big Data landscape by developing and offering solutions that help businesses use all their data (e.g., operational, constituency, financial) to improve the customer experience and optimize customer loyalty.
[…] [3] Vgl. Hayes, Bob: Advances, 2012. […]
[…] are three broad implications of Big Data to the field of customer experience management: 1) You can now ask/answer bigger questions; 2) You […]
[…] that we need to use more objective metrics of customer loyalty whenever they are available. Using Big Data principles, companies can link real loyalty behaviors with customer satisfaction ratings. Using a […]
[…] Care) were housed in separate databases on the data.medicare.gov site. As explained elsewhere in my post on Big Data, I linked these three data sets together by hospital name. Basically, I federated the necessary […]